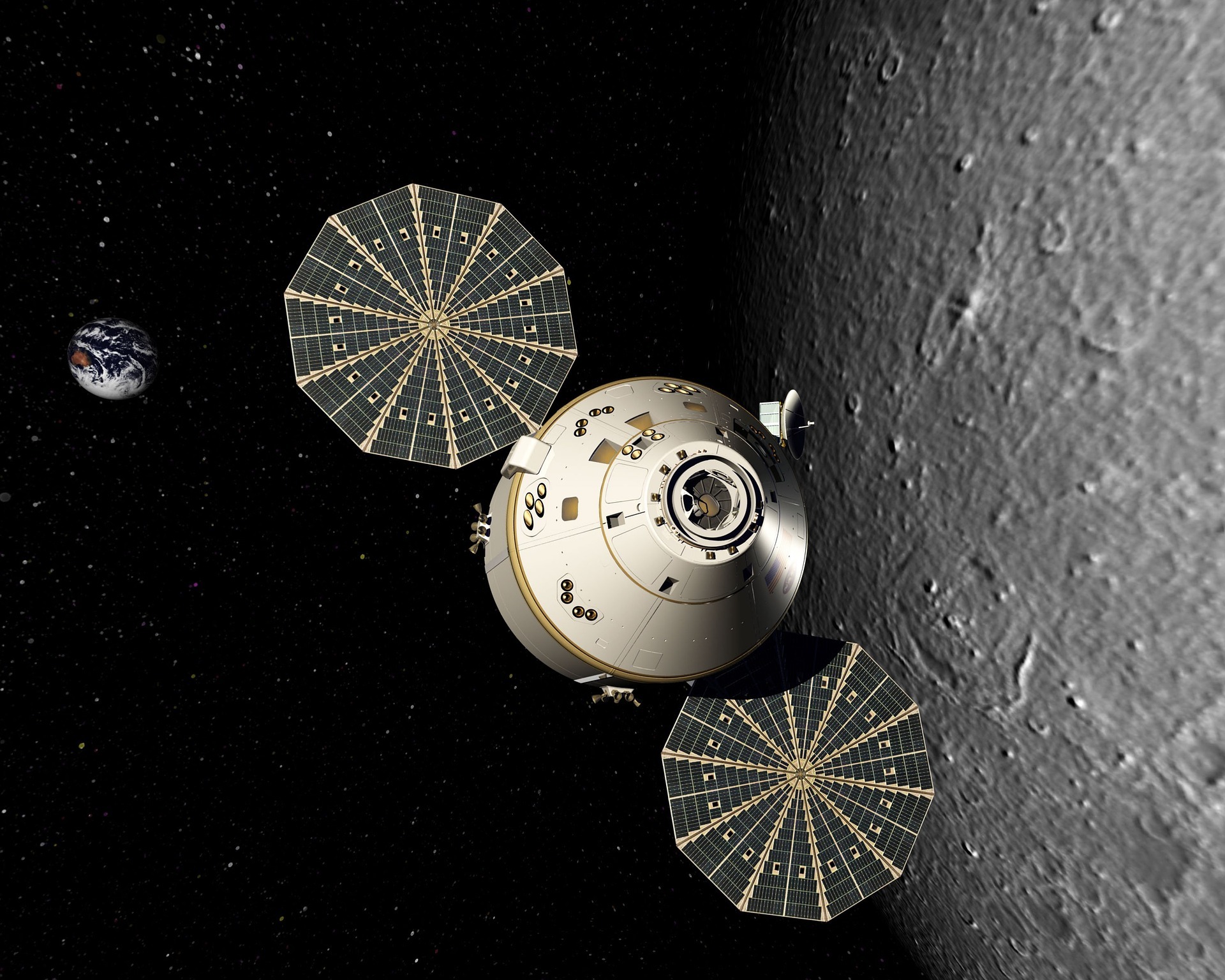
SAFEXPLAIN
PROJECT SUMMARY
SAFEXPLAIN is a project in the Horizon 2020 program that focuses on providing a novel and flexible approach to allow the certification and adoption of DL-based solutions in CAIS (Critical Autonomous AI-based Systems). It aims to do so by (1) architecting transparent DL solutions and libraries that allow explaining why they satisfy FUSA (Functional Safety) requirements, and by (2) devising alternative and increasingly complex FUSA design safety patterns for different DL usage levels, for varying levels of criticality and fault tolerance. The consortium is composed of research centers such as RISE (AI expertise), Ikerlan (FUSA expertise), and BSC (platform/performance expertise), together with companies in the fields of automotive (Navinfo), space (AIKO), railway (Ikerlan), and functional safety (Exida).
PROBLEM WE SOLVED
The project tackles applications in different critical scenarios, all employing DL to provide the operating systems with autonomy. The case studies selected as testbeds come from the industrial partners: the space sector, with an algorithm for autonomous navigation; automotive, with an algorithm for pedestrian identification and avoidance in the scope of autonomous driving; and railway, with an algorithm on obstacles detection on the rails and response. For every application, the safety criticality is evaluated and explainability tools are provided to enable a better understanding of the underlying functioning.
WHY IT IS IMPORTANT
SAFEXPLAIN explores the domain of safety, explainability, and validation of AI and DL-based algorithms, which is still quite uncovered by current standards and software assurance guidelines. The output will include both guidelines and safety patterns, which could be integrated into standards about AI verification and validation, and practical libraries to embed explainable and traceable features into a new generation of DL algorithms