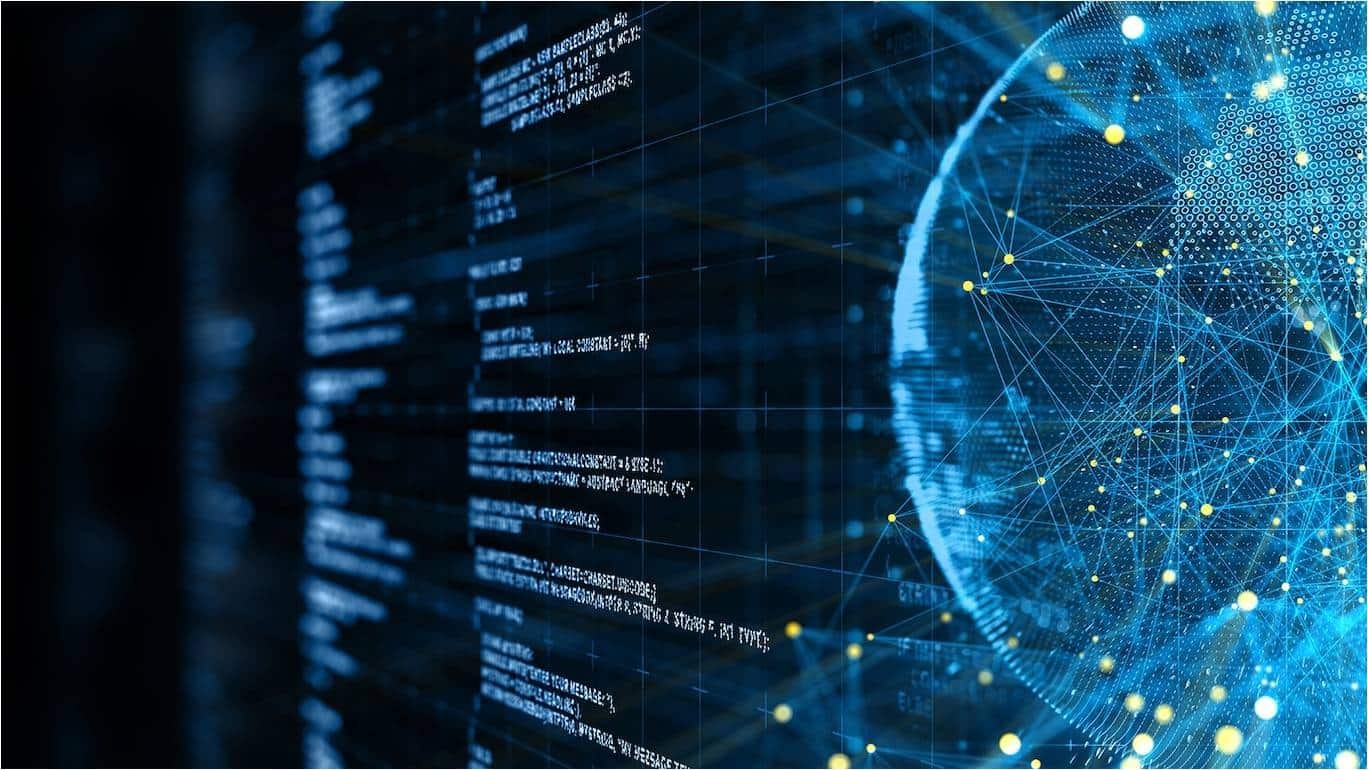
SMO
PROJECT SUMMARY
SMO (Spacecraft System and Subsystems models optimization by AIT/AIV and Operations big data analysis) is an ESA-funded activity that deals with the design, development, and demonstration of a software framework to ease the development and use of Machine Learning and Deep Learning algorithms in spacecraft AIV activities. The activity was carried out together with Altec, Thales Alenia Space, and SATE (Systems and Advanced Technologies Engineering).
PROBLEM WE SOLVED
The main objectives of the activities have been to exploit and analyze data generated from the design, integration, validation, and operation phases to create accurate Machine Learning models for systems and subsystems' lifecycle steps. Additionally, the developed framework can be used to support and optimize the specification, evolution, and maintenance of systems and subsystems and to enable Spacecraft engineers who are not data scientist experts to effectively exploit the benefits brought by the usage of Machine Learning models.
WHY IT IS IMPORTANT
The final product reached at the end of the activity was a Minimum Viable Product (MVP) with a TRL 6. This product can be used to ingest, decode/parse and pre-process Euclid data and to define, train and use Machine Learning models. In this context, AIKO worked on two use cases: the first one was aimed at training an ML model to support the K-band downlink selection to be adopted in order to overcome the adverse effect of transmission; the second application aimed at recognizing the optimality of the sequence of the files to download to raise a warning in case of sub-optimal sequences.
Publications
BIG DATA FROM SPACE 2021 "Spacecraft System and Subsystems models optimization by AIT/AIV and Operations big data analysis"